Artificial intelligence (AI) is a rapidly growing revolution with the potential to change and create many industries and the way things are done. But what impact will AI have on supply chain planning?
By Juan Pablo Mendez
Partner and Senior Project Manager, Miebach Chile
Before discussing these impacts, let's first put things into context: What does supply chain planning mean? At Miebach, we propose the following definition:
Supply Chain Planning = Decision-making process + balancing market requirements with our operational buffers
A decision-making process within the context of planning the supply chain refers to how we coordinate future actions within the value chain. Balancing market requirements with our operational buffers involves providing tailored information from the past that allows for projecting future performance.
Our operational buffers address questions such as: What stock level do I need to meet future projections (work, safety, WIP)? Can I modify my supply lead times to meet the required demand (by sea or air, domestic or import)? Can I offer a differentiated service level by sales channel to meet my requirements?
On the other hand, experience, within the context of planning, can be defined as follows:
Experience = Pattern recognition + Self-referencing
Pattern recognition involves understanding relationships between inputs and outputs through various activities. Self-referencing involves linking previous experiences with current situations to make informed decisions.
With these definitions in mind, we can establish the following relationship between supply chain planning, experience, and AI support in an advanced planning software (APS).
It is worth noting that, in a certain way, AI has been present in supply chain planning for a long time in the form of various heuristics that allow predicting future behavior to support decision-making. Today, with Deep Learning models like Chat GPT, the amount of data to process and the variables to consider have massively increased, which APS systems are incorporating.
One of the benefits of AI in APS is that there are few "hallucinations" (a term referring to an AI response that lacks coherence within the requested context), given that models tend to calibrate themselves based on the amount of repetitive data they can capture from the company’s systems (ERP, WMS, TMS, etc.). For example, Forecast vs. Sales, Plant Productivity vs. Production Mix, Distribution Center Productivity vs. Order Structure, among others, for "n" periods (days over "n" years). The tokens (number of variables, a term used in AI) generally remain consistent over time, allowing models to self-correct.
When it comes to production scheduling, the use of AI is excellent (given the repetitive nature of the tasks), as long as no unexpected events occur, such as a fire at the plant or a critical failure in a production line, where it becomes necessary to adjust parameters and/or rely on the experience of the planner/scheduler for decision-making.
External indicators that can complement the company's data (such as inflation, sporting events, exchange rates, weather, to name a few) and information from the physical world that can feed data into the APS (e.g., sensors) are challenges for AI support in APS that are currently being addressed.
The functions of the supply chain planner in a world infused with AI will not necessarily free them from work; while tasks such as data management and heuristic construction will be strongly supported by AI, the role of the "firefighter" (problem solver) and communication within the organization will increase as more tools become available for decision-making.
In the meantime, to implement these AI models (in conjunction with APS) within organizations, the tasks of data management and the construction of models that allow simulating and projecting requirements (heuristics) will be even more necessary to gain confidence in the results and "free" oneself from these areas.
Thus, the question is the degree of value that the planner will bring within this AI-infused world. Will there be "analysis paralysis" or overconfidence in the results provided by the models? This is one of the questions that arises from this analysis.
What we do know is that in a world—whether with or without AI—the ABC of prioritizing the implementation of people, processes, and systems will continue to be valid.
Therefore, we invite you to review your processes and how teams interact within your organization and systems to unleash the full potential of your supply chain.
For more information, let’s talk at: latinoamerica@miebach.com
Artificial Intelligence in the Context of Supply Chain Planning
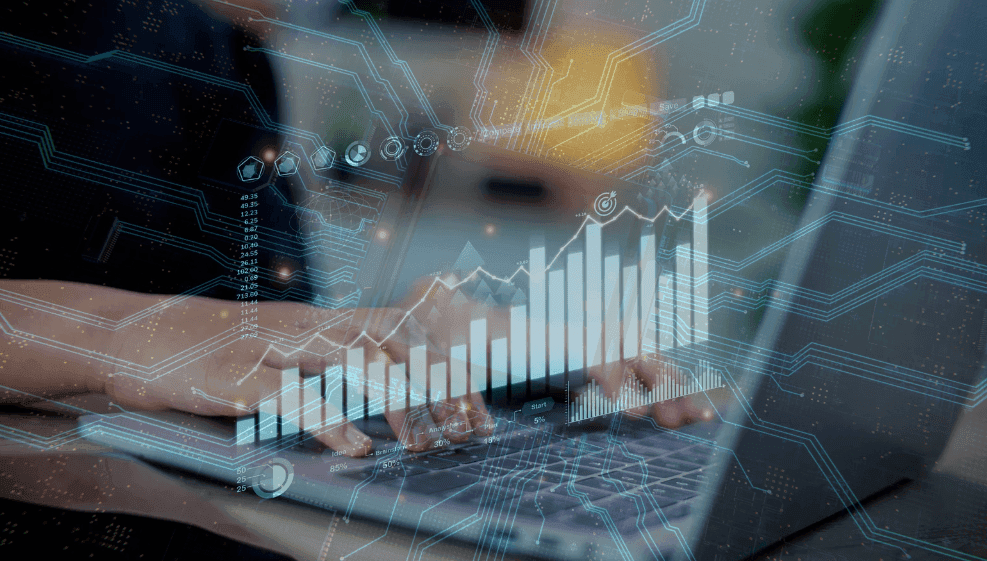